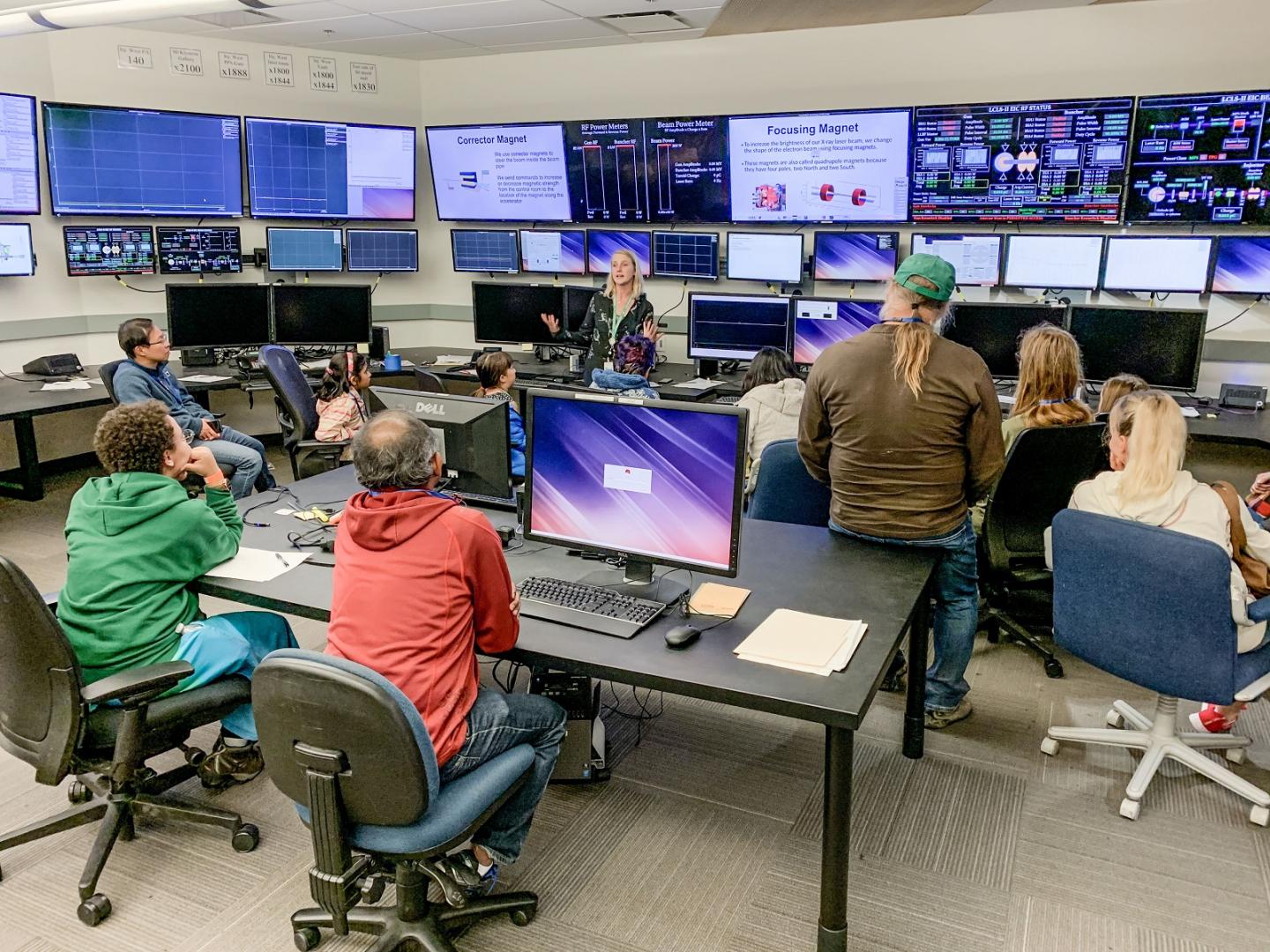
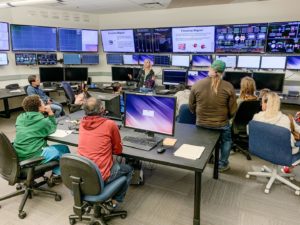
CREDIT
Jacqueline Orrell/SLAC National Accelerator Laboratory
Each year, researchers from around the world visit the Department of Energy’s SLAC National Accelerator Laboratory to conduct hundreds of experiments in chemistry, materials science, biology and energy research at the Linac Coherent Light Source (LCLS) X-ray laser. LCLS creates ultrabright X-rays from high-energy beams of electrons produced in a giant linear particle accelerator.
Experiments at LCLS run around the clock, in two 12-hour shifts per day. At the start of each shift, operators must tweak the accelerator’s performance to prepare the X-ray beam for the next experiment. Sometimes, additional tweaking is needed during a shift as well. In the past, operators have spent hundreds of hours each year on this task, called accelerator tuning.
Now, SLAC researchers have developed a new tool, using machine learning, that may make part of the tuning process five times faster compared to previous methods. They described the method in Physical Review Letters on March 25.
Tuning the beam
Producing LCLS’s powerful X-ray beam starts with the preparation of a high-quality electron beam. Some of the electrons’ energy then gets converted into X-ray light inside special magnets. The properties of the electron beam, which needs to be dense and tightly focused, are a critical factor in how good the X-ray beam will be.
“Even a small difference in the density of the electron beam can have a huge difference in the amount of X-rays you get out at the end,” says Daniel Ratner, head of SLAC’s machine learning initiative and a member of the team that developed the new technique.
The accelerator uses a series of 24 special magnets, called quadrupole magnets, to focus the electron beam similarly to how glass lenses focus light. Traditionally, human operators carefully turned knobs to adjust individual magnets between shifts to make sure the accelerator was producing the X-ray beam needed for a particular experiment. This process took up a lot of the operators’ time – time they could spend on other important tasks that improve the beam for experiments.
A few years ago, LCLS operators adopted a computer algorithm that automated and sped up this magnet tuning. However, it came with its own disadvantages. It aimed at improving the X-ray beam by making random adjustments to the magnets’ strengths. But unlike human operators, this algorithm had no prior knowledge of the accelerator’s structure and couldn’t make educated guesses in its tuning that might have ultimately led to even better results.
This is why SLAC researchers decided to develop a new algorithm that combines machine learning – “smart” computer programs that learn how to get better over time – with knowledge about the physics of the accelerator.
“The machine learning approach is trying to tie this all together to give operators better tools so that they can focus on other important problems,” says Joseph Duris, a SLAC scientist who led the new study.
A better beam, faster
The new approach uses a technique called a Gaussian process, which predicts the effect a particular accelerator adjustment has on the quality of the X-ray beam. It also generates uncertainties for its predictions. The algorithm then decides which adjustments to try for the biggest improvements.
For example, it may decide to try a dramatic adjustment whose outcome is very uncertain but could lead to a big payoff. That means this new, adventurous algorithm has a better chance than the previous algorithm of making the tweaks needed to create the best possible X-ray beam.
The SLAC researchers also used data from previous LCLS operations to teach the algorithm which magnet strengths have typically led to brighter X-rays, giving the algorithm a way of making educated guesses about the adjustments it should try. This equips the algorithm with knowledge and expertise that human operators naturally have, and that the previous algorithm lacked.
“We can rely on that physics knowledge, that institutional knowledge, in order to improve the predictions,” Duris says.
Insights into the magnets’ relationships to each other also improved the technique. The quadrupole magnets work in pairs, and to increase their focusing power, the strength of one magnet in a pair must be increased while the other’s is decreased.
With the new process, tuning the quadrupole magnets has become about three to five times faster, the researchers estimate. It also tends to produce higher-intensity beams than the previously used algorithm.
“Our ability to increase our tuning efficiency is really, really critical to being able to deliver a beam faster and with better quality to people who are coming from all over the world to run experiments,” says Jane Shtalenkova, an accelerator operator at SLAC who worked with Duris, Ratner and others to develop the new tool.
Beyond LCLS
The same method can be extended to tune other electron or X-ray beam properties that scientists may want to optimize for their experiments. For example, researchers could apply the technique to maximize the signal they get out of their sample after it’s hit by LCLS’s X-ray beam.
This flexibility also makes the new algorithm useful for other facilities.
“The nice thing about this machine learning algorithm is that you can do tech transfer relatively easily,” says Adi Hanuka, a SLAC scientist who has been testing the technique at three other accelerators: SPEAR3, the accelerator ring powering SLAC’s Stanford Synchrotron Radiation Lightsource (SSRL); PEGASUS at the University of California, Los Angeles; and the Advanced Photon Source (APS) at DOE’s Argonne National Laboratory.
“This tool now exists in several labs,” Hanuka says. “Hopefully, we’ll be integrating it into even more labs soon.”